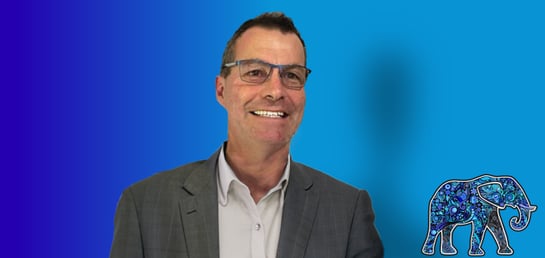
PostgreSQL's B-Tree indexing system is built on multiple layers of optimizations, each addressing different challenges in concurrency, storage efficiency, and performance. These optimizations stack together, creating a highly efficient and scalable indexing mechanism.
Layer 1: The Lehman & Yao algorithm for high concurrency
At the foundation of PostgreSQL's B-Tree implementation is the Lehman & Yao algorithm, which optimizes concurrent access in multi process/threaded environments. This algorithm introduces the following features to reduce lock contention during node splits and updates:
- Right links between nodes, allowing readers to traverse the tree without being blocked by ongoing updates
- High keys for non-blocking page splits, enabling concurrent inserts without degrading read performance
Layer 2: B+ Tree structure for efficient storage and range queries
Building on Lehman & Yao's concurrency optimizations, PostgreSQL implements B+ Trees, an improved version of traditional B-Trees. The key differences that make B+ Trees more efficient include:
- Leaf nodes store all actual data indexed, while internal nodes contain only keys, reducing index size and improving cache efficiency.
- Linked leaf nodes (pointers going in both directions between adjoining leaf nodes), which allows PostgreSQL to efficiently scan larger result sets particularly for range queries and sorted results
- Higher branching factors, meaning shallower trees and fewer disk I/O operations per lookup
This structure enables PostgreSQL to handle large amounts of data efficiently, making range-based queries and index scans much faster than if a standard B-Tree structure was used.
Layer 3: PostgreSQL-specific optimizations for performance and storage efficiency
PostgreSQL further enhances its B+ Tree implementation with several additional optimizations:
- HOT (Heap-Only Tuple) updates
- Index deduplication
- B-Tree page deletion
- Parallel index scans
- Index-only scans
- Covered indexes
Let's take a closer look at each of them separately.
HOT (Heap-Only Tuple) updates
The Heap-Only Tuple updates feature has been a relatively long-standing optimization for PostgreSQL B-Tree indexes, with its implementation in version 8.3. The feature avoids unnecessary index updates where non-indexed columns are modified on a tuple in the heap. This has the benefit of reducing bloat in the index, lower disk I\O, and less frequent vacuuming and reindexing.
PostgreSQL's Multi-Version Concurrency Control (MVCC) system is designed to reduce locking, by ensuring that updates do not overwrite rows that might still be required by an older transaction, by creating a new row version tuple in the heap table. When a new tuple is created in the same page in this way, the old tuple pointer (CTID) is re-directed to point to the new tuple, meaning that there is no need to create a new index entry as would be the case in a traditional approach. The old tuple is then marked as dead on the heap, ready for cleaning up later.
Because we do not need to modify the index, we help reduce index bloat by avoiding redundant index inserts.
Let's explore this some more in PSQL using the boat table and the boat_idx1 index.
We can see our B-Tree index only includes the id column from the boat table.
bt=# \d boat
Table "public.boat"
Column | Type | Collation | Nullable | Default
--------+------------------+-----------+----------+---------
id | integer | | |
name | text | | |
reg | double precision | | |
Indexes:
"boat_idx1" btree (id)
bt=# \d boat_idx1
Index "public.boat_idx1"
Column | Type | Key? | Definition
--------+---------+------+------------
id | integer | yes | id
btree, for table "public.boat"
That means that if we update the name or reg columns, we don't want to see the index updated. So, if we update the name column on the row with id=345, and then query the pg_stat_all_tables catalogue table (which collects ongoing statistics about tables in the database), we will be able to see if this update used this feature. All we need to do is check the columns n_tup_hot_upd, which counts the number of Heap-Only Tuple (HOT) updates for each table, and n_tup_upd, which counts the total number of updates to the table.
bt=# UPDATE boat SET name = 'trade winds' WHERE id=345;
UPDATE 1
bt=# SELECT n_tup_hot_upd, n_tup_upd, relname FROM pg_stat_all_tables WHERE relname = 'boat';
n_tup_hot_upd | n_tup_upd | relname
---------------+-----------+---------
0 | 1 | boat
(1 row)
And above we can see that we didn't do a HOT update. Why is that?
It is because when we created the table, we left the fillfactor set to the default of 90%. This means that we fully utilize each page, filling it up with tuples so that there are no gaps. The HOT update feature requires the reference to be updated to point to a new tuple on the same page. In this case there is no room on the same page, so the HOT update feature could not be used.
We can set the fillfactor option to leave gaps in each page by using the with option in our CREATE TABLE command. However, while this might make updates more efficient, it means that our rows will be spread out over more pages, making operations like sequential scans more time-consuming. So this setting needs to be used carefully with an understanding of how the table will be used.
Let's create a new table called boat2, with a fillfactor setting of 90% using our boat table as a source. We'll then create the same index on the id column, allowing us to try the same update and see if the HOT update feature is used in this case.
bt=# CREATE TABLE boat2 WITH (fillfactor=90) AS SELECT * FROM boat;
SELECT 504500
bt=# \d boat2
Table "public.boat2"
Column | Type | Collation | Nullable | Default
--------+------------------+-----------+-----------+----------
id | integer | | |
name | text | | |
reg | double precision | | |
bt=# CREATE INDEX boat2_idx ON boat2(id);
CREATE INDEX
We can check what options have been set on a table by querying the pg_class table. The column we are after is called reloptions (short for relation options), and we can use relname to filter the results using tablename. In the below example, we can see that our fillfactor is set to 90% as expected. If the default was used, there would not be any options returned.
bt=# SELECT c.relname AS "name", c.reloptions AS "options" FROM pg_class AS C WHERE c.relname = 'boat2';
name | options
-------+---------------
boat2 | {fillfactor=90}
(1 row)
Let's check that statistics are initialized for our new table. Then, let's apply the same UPDATE command, followed by requiring the statistics table.
bt=# SELECT n_tup_hot_upd, n_tup_upd, relname FROM pg_stat_all_tables WHERE relname = 'boat2';
n_tup_hot_upd | n_tup_upd | relname
---------------+-----------+---------
0 | 0 | boat2
(1 row)
bt=# UPDATE boat2 SET name = 'Fair Lands' where ID = 346;
UPDATE 1
bt=# SELECT n_tup_hot_upd, n_tup_upd, relname FROM pg_stat_all_tables WHERE relname = 'boat2';
n_tup_hot_upd | n_tup_upd | relname
---------------+-----------+---------
1 | 1 | boat2
(1 row)
bt=# UPDATE boat2 SET name = 'Fair Lands' WHERE id > 346 AND id < 1000;
UPDATE 1152
bt=# SELECT n_tup_hot_upd, n_tup_upd, relname FROM pg_stat_all_tables WHERE relname = 'boat2';
n_tup_hot_upd | n_tup_upd | relname
---------------+-----------+---------
226 | 1153 | boat2
(1 row)
We can see that we have done a HOT update this time, as the n_tup_hot_upd count has also been incremented.
Let's now go ahead and update lots of rows and look at the effect. We can see that we have updated 1152 rows (we have duplicate ids, as this column does not have a unique constraint set), but only 226 of them were HOT updates.
This is because the update we did updated many tuples on the same database page, and there was only enough spare room on the page (with a fillfactor of 90% - 10% space) to update some of the tuples this way.
So, while this is a great optimization, you need to be very careful about how you utilize it.
Index deduplication
Introduced in PostgreSQL 13, this feature reduces an index's size where repeated values occur.
We saw in the previous example on HOT updates that we had duplicate values in our indexed column id. Prior to version 13 of PostgreSQL, each value required a separate index entry, even if it appeared twice. The deduplication feature combines identical index entries into a single index entry, contributing to a smaller index size, I/O efficiency, and faster index scans.
We can use the query SELECT * FROM bt_page_items(get_raw_page('boat_idx1',2)) function that we used in the previous article to look at our index entries for the boat table and look at the entries where there are duplicate ids:
229 | (4715,90) | 16 | f | f | f2 01 00 00 00 00 00 00 | f | (4715,90) | 230 | (4,71) | 16 | f | f | f3 01 00 00 00 00 00 00 | f | (4,71) | 231 | (4715,91) | 16 | f | f | f3 01 00 00 00 00 00 00 | f | (4715,91) | 232 | (4,72) | 16 | f | f | f4 01 00 00 00 00 00 00 | f | (4,72) | 233 | (4715,92) | 16 | f | f | f4 01 00 00 00 00 00 00 | f | (4715,92) | 234 | (16,8194) | 32 | f | f | f5 01 00 00 00 00 00 00 | f | (4,73) | {"(4,73)","(46,79)"} 235 | (4715,93) | 16 | f | f | f5 01 00 00 00 00 00 00 | f | (4715,93) | 236 | (4715,94) | 16 | f | f | f5 01 00 00 00 00 00 00 | f | (4715,94) | 237 | (16,8194) | 32 | f | f | f6 01 00 00 00 00 00 00 | f | (4,74) | {"(4,74)","(46,80)"} 238 | (4715,95) | 16 | f | f | f6 01 00 00 00 00 00 00 | f | (4715,95) | 239 | (4715,96) | 16 | f | f | f6 01 00 00 00 00 00 00 | f | (4715,96) | 240 | (16,8194) | 32 | f | f | f7 01 00 00 00 00 00 00 | f | (4,75) | {"(4,75)","(46,81)"} 241 | (4715,97) | 16 | f | f | f7 01 00 00 00 00 00 00 | f | (4715,97) | 242 | (4715,98) | 16 | f | f | f7 01 00 00 00 00 00 00 | f | (4715,98) | 243 | (16,8194) | 32 | f | f | f8 01 00 00 00 00 00 00 | f | (4,76) | {"(4,76)","(46,82)"} 244 | (4715,99) | 16 | f | f | f8 01 00 00 00 00 00 00 | f | (4715,99) | 245 | (4715,100) | 16 | f | f | f8 01 00 00 00 00 00 00 | f | (4715,100) | 246 | (16,8194) | 32 | f | f | f9 01 00 00 00 00 00 00 | f | (4,77) | {"(4,77)","(46,83)"} 247 | (4715,101) | 16 | f | f | f9 01 00 00 00 00 00 00 | f | (4715,101) | 248 | (4715,102) | 16 | f | f | f9 01 00 00 00 00 00 00 | f | (4715,102) | 249 | (16,8194) | 32 | f | f | fa 01 00 00 00 00 00 00 | f | (4,78) | {"(4,78)","(46,84)"} 250 | (4715 103) | 16 | f | f | fa 01 00 00 00 00 00 00 | f | (4715,103) |
We can see in the above screenshot that those index entries where there are duplicate keys - they are listed with gray background against a single entry.
B-Tree page deletion
Introduced in PostgreSQL 14, this feature automatically removes unused pages from indexes, reducing bloat. Before this feature's implementation, deleted rows were marked as dead. Even if all tuples in an index page were deleted and marked dead, the pages remained a part of the index, taking up space (bloat).
The only way to reclaim this space was to manually rebuild the index using the REINDEX command.
After implementation of this feature, PostgreSQL automatically removes empty pages when the following conditions are met:
- The page is not a root or internal page
- No active transactions reference the page
- A neighbouring sibling page exists to redistribute pointers
While automatic index page deletion might be enabled, it does not always work, due to several reasons such as transaction visibility, structural constraints, rebalancing behaviour, and autovacuuming delays. Therefore, if automatic deletion does not occur as expected, manually forced vacuuming or a rebuild of the index may be required.
Should a table require frequent delete, it may be worth considering a strategy that involves partitioning or partial indexes for improved efficiency.
Parallel index scans
Parallel index scans were introduced in PostgreSQL 11, and allow multiple workers to handle a separate chunk of index. The results are combined and returned faster than the previous single-threaded index scan.
There is an overhead for management of splitting an index scan across multiple processes, so the table needs to be large enough to justify parallel execution. The instance must also have sufficient worker processes available to support it.
Let's try it out with our boat table and its index. First, let's make sure we will have enough parallel workers for our query, and lower the cost parameters to improve the chance of a parallel index scan being used.
bt=# ALTER SYSTEM SET max_parallel_workers_per_gather = 6;
ALTER SYSTEM
bt=# ALTER SYSTEM SET parallel_tuple_cost = 0.1;
ALTER SYSTEM
bt=# ALTER SYSTEM SET parallel_setup_cost = 50;
ALTER SYSTEM
Next, we will insert enough rows to offset the overhead. 3 million rows should be enough.
bt=# INSERT INTO boat(id, name, reg) SELECT 1, md5(1::text), log(1) FROM generate_series(1,3000000) AS t;
INSERT 0 3000000
bt=# SELECT COUNT(id) FROM boat;
count
---------
3000000
(1 row)
Then, we will do an EXPLAIN ANALYZE to see how the planner decides to do a range query that should cause 2/3 of the index to be scanned.
bt=# EXPLAIN ANALYZE SELECT SUM(id) FROM boat WHERE id > 2000 AND id < 2000000;
QUERY PLAN
-------------------------------------------------------------------------------------
Finalize Aggregate (cost=49864.99..49865.00 rows=1 width=8) …
-> Gather (cost=49864.78..49864.99 rows=2 width=8) …
Workers Planned: 2
Workers Launched: 2
-> Partial Aggregate (cost=48864.78..48864.79 rows=1 width=8) …
-> Parallel Seq Scan on boat (cost=0.00..46788.00 rows=830712 width=4) …
Filter: ((id > 2000) AND (id < 2000000))
Rows Removed by Filter: 334000
Planning Time: 3.052 ms
Execution Time: 141.988 ms
(10 rows)
Unfortunately, this resulted in a parallel sequential scan of the heap pages (still more efficient than a single threaded sequential scan). This is likely due to the larger range we specified. So, let's reduce our upper end of the range down to 200000 and see what happens.
bt=# EXPLAIN ANALYZE SELECT SUM(id) FROM boat WHERE id > 2000 AND id < 200000;
QUERY PLAN
-------------------------------------------------------------------------------------------------------------
Finalize Aggregate (cost=6442.53..6442.54 rows=1 width=8) (actual time=26.990..27.045 rows=1 loops=1)
-> Gather (cost=6442.31..6442.52 rows=2 width=8) (actual time=26.984..27.041 rows=3 loops=1)
Workers Planned: 2
Workers Launched: 2
-> Partial Aggregate (cost=5442.31..5442.32 rows=1 width=8) (actual time=20.459..20.469 rows=1 loops=3)
-> Parallel Index Only Scan using boat_idx1 on boat (cost=6.43..5237.25 rows=82624 width=4) …
Index Cond: ((id > 2000) AND (id < 200000))
Heap Fetches: 8
Planning Time: 0.194 ms
Execution Time: 27.082 ms
(10 rows)
This time, a parallel index scan was used with 2 workers dividing the task between them.
Typically, you will find that parallel scans provide benefits when you have large tables with millions of rows, range-based queries, and aggregation queries, all of which are typically found in OLAP-style workloads (so this is a good indicator; see if you can adjust settings to get better performance by inducing a parallel index scan).
Index-only scans
This feature enables queries to fetch data directly from indexes without accessing the heap. Traditionally, an index scan will retrieve row pointers from the index, but then must follow them to the heap to retrieve the selected row data. Since PostgreSQL 9.2, PostgreSQL will store the values in the index, avoiding the heap lookup. This significantly improves query performance with far less disk access. But it only works if all of the SELECT columns are in the index.
We saw in the previous section on parallel index-only scans that only the index needs to be used when we selected the id column. Let's now select another column that isn't stored on the index and see what happens:
bt=# EXPLAIN ANALYZE SELECT id, name FROM boat WHERE id > 2000 AND id < 200000;
QUERY PLAN
-------------------------------------------------------------------------------
Index Scan using boat_idx1 on boat (cost=0.43..8228.59 rows=196858 width=37) …
Index Cond: ((id > 2000) AND (id < 200000))
Planning Time: 1.182 ms
Execution Time: 84.548 ms
(4 rows)
This time we can see that by including name in the query, that the planner performs an index scan instead of an index-only scan, where it needs to access the heap to read the name for each row.
Covered indexes
Covered indexes (or covering indexes) are indexes that contain all the columns needed to execute a query, allowing PostgreSQL to perform an index-only scan. We can add the additional columns needed without making them a part of the index using the INCLUDE keyword:
bt=# CREATE INDEX boat_idx_covered ON boat(id) INCLUDE (name);
CREATE INDEX
bt=# EXPLAIN ANALYZE SELECT id, name FROM boat WHERE id > 2000 AND id < 200000;
QUERY PLAN
--------------------------------------------------------------------------------
Index Scan using boat_idx1 on boat (cost=0.43..8228.59 rows=196858 width=37) …
Index Cond: ((id > 2000) AND (id < 200000))
Planning Time: 2.215 ms
Execution Time: 46.473 ms
(4 rows)
bt=# DROP INDEX boat_idx1;
DROP INDEX
bt=# EXPLAIN ANALYZE SELECT id, name FROM boat WHERE id > 2000 AND id < 200000;
QUERY PLAN
--------------------------------------------------------------------------------------------
Index Only Scan using boat_idx_covered on boat (cost=0.43..9585.59 rows=196858 width=37) …
Index Cond: ((id > 2000) AND (id < 200000))
Heap Fetches: 0
Planning Time: 0.432 ms
Execution Time: 44.123 ms
(5 rows)
Notice that even after we created the new covered index, the planner still used the old index and performed an ordinary index scan. But once we dropped the old index, the new one was used to perform an index-only scan.
We don't need the old index, as the new boat_idx_covered index will also do the job of the first index. So, it is worth being aware of this when planning what indexes you need.