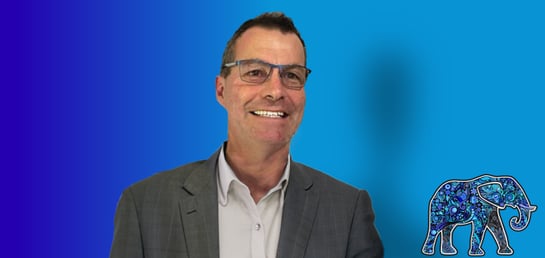
A new era for Fujitsu Enterprise Postgres
With the release of Fujitsu Enterprise Postgres 17, pgvector support is on the horizon, introducing efficient vector operations and similarity searches within the PostgreSQL environment.
Additionally, PostgresML is set to be supported in Service Pack 1, bringing in-database machine learning capabilities. With these advancements, now is a great time to examine the types of solutions these extensions will enable and how they address some of today’s data challenges.
This article looks at how pgvector and PostgresML can empower organizations to tackle problems ranging from classification and regression to natural language processing and recommendation systems. Together, they aim to transform Fujitsu Enterprise Postgres into a powerful tool for data science, allowing businesses to gain insights faster and at a lower cost by consolidating ML and vector capabilities within a single, unified platform.
PostgresML: Enabling in-database ML for classification, regression, and clustering
Classification problems
Classification tasks, where data is categorized into distinct classes, are widely used in areas like fraud detection, sentiment analysis, and customer segmentation. PostgresML makes it possible to perform these tasks directly in FEP by training and deploying classification models within the database. By doing so, users eliminate the need to export data to separate ML environments, which streamlines the process and reduces latency.
Regression for forecasting and predictive analytics
Regression is essential for predictive analytics, helping organizations forecast trends such as sales, customer growth, and resource needs. With PostgresML, users can apply regression techniques on their data, enabling predictions directly within Fujitsu Enterprise Postgres and supporting data-driven decisions without relying on external ML tools.
Clustering for customer segmentation and anomaly detection
Clustering is a popular technique for segmenting customers or detecting anomalies in data. With PostgresML, clustering models can be implemented in-database, which is ideal for applications that need to group data points dynamically without needing specialized environments.
Revolutionizing NLP, recommendations, and similarity searches with vectors
Natural Language Processing (NLP) for semantic search
pgvector’s support for vector embeddings makes it a powerful tool for NLP tasks. By storing text embeddings - numerical representations of words or phrases - pgvector enables semantic search within Fujitsu Enterprise Postgres, where users can retrieve similar documents or perform context-aware searches.
This is essential for applications that require understanding the meaning behind user queries, rather than relying solely on keyword matches.
Recommendations through vector-based similarity matching
Recommendations often rely on matching items or users based on their vectorized attributes, such as preferences, behaviours, or item characteristics. With pgvector, these vectors can be stored and searched within Fujitsu Enterprise Postgres, enabling recommendation engines without the need for external vector databases.
Image and audio similarity searches
Beyond NLP, pgvector can also handle embeddings for multimedia data like images or audio. This makes it ideal for applications that need to perform similarity searches across different media types, allowing for innovative, cross-platform experiences.
How PostgresML and pgvector address key data science needs
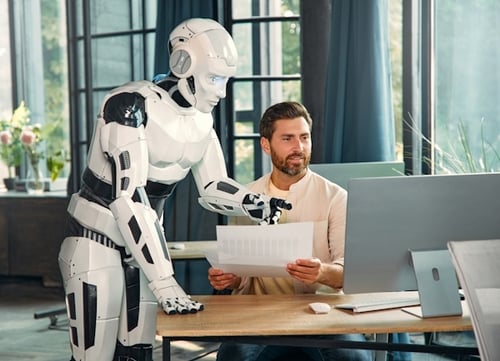
- Unified workflow
By embedding ML and vector operations within Fujitsu Enterprise Postgres, PostgresML and pgvector eliminate the need for specialised environments, simplifying data workflows and lowering infrastructure costs. - Reduced latency
Real-time applications benefit from the low-latency environment provided by in-database ML and vector similarity searches, essential for quick responses in fraud detection, recommendations, and more. - Cost-efficiency
Reducing reliance on external tools for ML and vector management brings down the total cost of ownership, making PostgreSQL a versatile, cost-effective solution for handling data science needs.
By offering native support for machine learning and vector operations, PostgresML and pgvector are set to transform Fujitsu Enterprise Postgres into a powerful, multi-functional database capable of solving classification, regression, NLP, and recommendation challenges directly within the data environment. This will allow organisations to accelerate their data initiatives while maintaining streamlined operations and reducing complexity in the ML lifecycle.