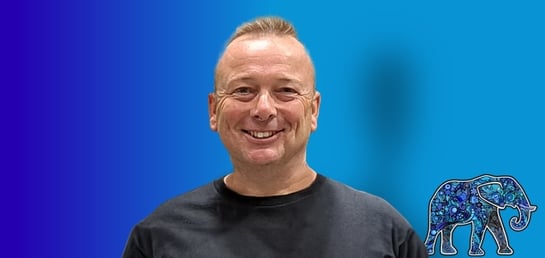
Optimizing enterprise databases: from default settings to dynamic scalability
Most enterprise databases come with fairly modest out-of-the box default parameter values. This approach offers basic functionality across operating systems and hardware specifications, without needing to know what the intended workloads are to be. DBAs then work with Application teams to reconfigure database parameters to closely align with known requirements, standards based on previous learnings, and expected usage.
After an application has been deployed, the database continues to evolve, with schema changes, code changes, database growth, database storage changes, fluctuating workloads, data ingestion changes, and data bloat being some of the characteristics of the database management lifecycle. It is important that databases can scale (vertically and/or horizontally) to meet shifts in volumes and activity.
Intelligent tuning with DBtune
DBtune introduces an AI OaaS (Optimizer As A Service) which offers real-time, automated, and pragmatic database tuning to optimize an instance based on the available hardware resources.
It does this by leveraging advanced ML (Machine Learning) to dynamically adapt and optimize database parameter settings. Which parameters it considers depends upon whether you choose to allow DBtune to restart the database instance as part of its optimization.
Parameter | Consider parameter if instance is allowed to restart |
Consider parameter if instance is not allowed to restart |
bgwriter_lru_maxpages | ||
checkpoint_completion_target | ||
effective_io_concurrency | ||
max_parallel_workers | ||
max_parallel_workers_per_gather | ||
max_wal_size | ||
max_worker_processes | ||
random_page_cost | ||
seq_page_cost | ||
shared_buffers | ||
work_mem |
The architecture involves a client running on the target host which monitors PostgreSQL and OS performance using pg_stat_statements & Python/psutil. All data collected is purely performance metrics, and stored into a vector database on the DBtune instance.
The capture will run 30 iterations, each lasting around 12 minutes before it will generate a suggested parameter change file under the conf.d folder. It does not alter the original postgresql.conf file, but parameters set in the conf.d folder will override anything in the postgresql.conf file.
The screenshot below shows a tuning run, and the 30 different points on the graph show the different tuning iterations it went through. As the output, it generated a configuration file that generated a 5.66 fold improvement.
Whilst it is recommended where possible to run DBtune against the target, it is possible to run it against a matching simulation environment with a similar workload, then export the tuning configuration and promote to a Production environment. This might be desirable if you want to choose the allow restart option to get the maximum benefit from the tuning run.
Advantages of the performance tuning
The potential gains from using DBtune include:
Reduced CapEx expenditure on CPU/RAM and storage
Faster response times
Reductions in unplanned downtime
Automation savings on OpEx
Shift from reactive to proactive monitoring