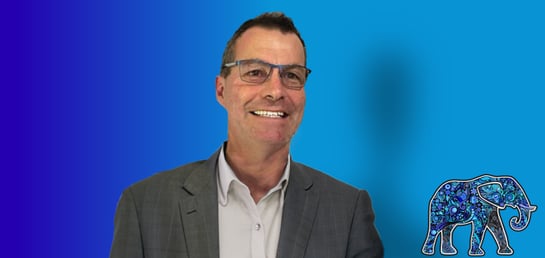
A new working model emerges
The COVID-19 pandemic has accelerated a paradigm shift towards remote and hybrid working models, fundamentally transforming the way organizations operate. These work models are predicted to not only become established, but to become more widely adopted globally.
This transition has introduced a myriad of challenges, particularly in optimizing the logistics of face-to-face meetings and other on-site collaborative activities, which are now often spread across various locations and time zones. These challenges will be further exacerbated by the increasing collaboration of teams across the globe.
Addressing these challenges necessitates a sophisticated route and scheduling optimization system. Such a system aims to determine optimal parameters including time, location, mode of transport, importance of attendees, and safety. By leveraging existing services and enhancing them through Artificial Intelligence and the use of graph databases, this system can streamline operations and foster a more efficient collaboration ecosystem. The goal is to integrate diverse data sources and services, creating a cohesive solution that intelligently evaluates and optimizes travel and meeting logistics.
Leveraging graph databases
A key component of this optimization framework is the use of graph databases. Graph databases excel in modelling and analyzing complex relationships and networks, making them particularly suited for route optimization tasks.
Apart from being proficient at calculating shortest paths, graph database are also great at evaluating various relational aspects, such as attendee availability, cost constraints, and transportation modes.
These capabilities extend graph databases use beyond mere pathfinding - they make them a powerful tool for uncovering and understanding intricate connections and dependencies within data, facilitating more informed and effective decision making. By capturing the multifaceted nature of logistical planning, graph databases enable the system to offer nuanced recommendations that align with organizational goals and constraints.
The intricacies of remote and hybrid working present challenges that require a comprehensive route optimization system, in which graph databases play a pivotal role.
Scoping the challenge
Hybrid work models have quickly become the standard arrangement for many knowledge workers, providing them with an enhanced work-life balance and increased job satisfaction. These arrangements grant workers greater control over the location and timing of their tasks, leading to improved flexibility, autonomy, and work-life balance.
Gallup surveyed a nationally representative sample of 8090 remote capable employees in the US and found that 60% now prefer hybrid work options.
While hybrid work models are acknowledged to have significant benefits for both organizations and their employees, there are significant challenges that must be addressed to realize these benefits.
The four commonly identified challenges include:
- Coordination and scheduling
Ensuring effective meeting coordination across different time zones and locations.
- Employee wellbeing
Addressing issues related to isolation, burnout, and maintaining a work-life balance.
- Technology and infrastructure
Implementing and maintaining the necessary technology and digital tools to support remote work.
- Management and communication
Developing new management practices to support remote teams and ensuring clear, effective communication.
Effective scheduling
Our solution aims to address the critical challenge of scheduling, using technology. Proper scheduling is essential to prevent overlapping meetings, missed deadlines, and a lack of coherence in project timelines.
Effective scheduling ensures that all team members can participate in meetings and collaborative sessions, reducing the risk of overwork and burnout by maintaining clear boundaries between work and personal life. It helps distribute workloads evenly, allowing employees adequate downtime and reducing stress associated with last-minute changes and urgent requests. Additionally, timely and well-coordinated meetings with clients and stakeholders are crucial for maintaining trust and ensuring the smooth progress of projects.
Effective scheduling accommodates the availability of external parties, leading to stronger client relationships and successful project outcomes.
Route optimization systems
Route optimization systems are designed to determine the most efficient paths or schedules for a variety of transportation and logistics operations. These graph-based systems leverage algorithms and data analysis to optimize travel time, fuel consumption, and operational costs, while optimizing efficiency and service quality.
Common applications include delivery route planning for logistics companies, ride-sharing services, and public transportation scheduling.
Current state
Incorporating existing route planning services into our collaboration solution offers several compelling advantages, particularly for creating a sustainable and efficient system that respects individual personal lives. These services have matured over time, becoming highly sophisticated and capable of handling the complex logistics of modern transportation and scheduling needs.
Existing route planning services like Route4Me, OptimoRoute, and Paragon Software Systems have already developed robust infrastructures capable of managing extensive data and optimized routes for various use cases.
These platforms have accumulated vast amounts of data from numerous clients, allowing them to fine-tune their algorithms and offer highly efficient solutions. By leveraging these established services, we can tap into a wealth of data and experience, ensuring that our solution benefits from the most accurate and optimized routing available. This is crucial for maintaining efficiency and optimal costs in our operations.
Route planning can be incredibly complex, involving multiple variables such as traffic conditions, vehicle capacities, delivery time windows, and varying transportation modes.
Services like Verizon Connect and Onfleet have integrated advanced algorithms, including Genetic Algorithms A genetic algorithm (GA) is a type of computational optimization technique inspired by the principles of natural selection and genetics. In a genetic algorithm, a population of candidate solutions to an optimization problem is evolved toward better solutions. and Ant Colony OptimizationAnt Colony Optimization (ACO) is a metaheuristic optimization technique inspired by the behavior of real ants when finding the shortest path to food sources. ACO algorithms solve complex optimization problems that can be represented as finding good paths through graphs, and are particularly effective for problems involving graph-based structures, such as vehicle routing, internet routing, and scheduling., to handle these complexities effectively. These platforms can dynamically adjust routes in real time, considering unforeseen changes like traffic jams or new delivery requests, which is essential for maintaining optimal performance.
By leveraging these advanced capabilities, our collaboration solution can ensure that all logistical challenges are managed seamlessly, enhancing overall efficiency and reliability.
Many modern route planning services now offer features that allow users to specify parameters such as transportation mode and calculate associated costs, both monetary and environmental. For example, OptimoRoute and Routific provide options to select between different transportation methods and assess their impact on the environment. This functionality is particularly important for developing a sustainable solution that optimizes carbon footprints and aligns with optimization goals for sustainability. By integrating these services, we can provide a solution that not only optimizes routes for cost effectiveness, but also promotes environmentally-friendly practices.
Options for smart scheduling
To create a system that respects individuals' personal lives, it is essential to have a flexible and intelligent scheduling component. Existing services can be configured to consider personal preferences and constraints, such as preferred working hours or specific days off. This level of optimization ensures that the scheduling system is not only efficient but also user-friendly, promoting better work-life balance and overall satisfaction among team members.
Among the best services to integrate are:
- Route4Me
Known for its scalability and ease of use, allowing for detailed route optimization and support for various transportation modes - OptimoRoute
Offers robust features for real-time traffic updates and environmental impact assessment, making it ideal for sustainable planning - Verizon Connect
Integrates real-time tracking and dynamic routing capabilities, enhancing operational flexibility, increasing worker productivity and safe driving - Routific
Optimizes in delivery route optimization with a strong emphasis on reducing operational costs and environmental impact
By leveraging these services, we can develop a highly effective, sustainable, and user-friendly route planning solution.
System goal
While we have discussed route optimization, the scheduling of an optimal location and time for collaboration is not directly dependent on calculating an optimal route. Individual attendees can determine the best route to the location using various available tools at the time of travel.
The purpose of this system is to identify the best location and time candidates based on median values of several configurable considerations for the person scheduling the meeting to select from. These considerations could be either mandatory, which includes safety of the person traveling, transit time, and environmental impact, or they could be optional, like whether other meetings scheduled around the same time at the same location should be factored in, or whether the location rates highly on an individual's preferences for the location at that time. Weightings can also be applied across these considerations to suit those areas most important to the person or system scheduling the meeting.
We do not need to know the best routes at the time of scheduling, but we do need to know which times and locations are most likely to offer a quick and convenient commute for individuals invited. This information is derived from existing route optimization services and can be pre-calculated and periodically updated across individual caches.
A distributed architecture is well-suited for this type of system, where independent responsibilities exist and can be managed by separate components. This approach offers several advantages, including scalability, reliability, and fault tolerance. It also provides flexibility and maintainability, performance optimization, cost efficiency, and an improved user experience.
Additionally, this architecture supports asynchronous processing and real-time updates, ensuring that the system remains responsive and efficient. By leveraging distributed computing, the system can handle large volumes of data and complex calculations, providing accurate and timely recommendations for scheduling optimal meeting times and locations.
Architecture
Distributed components
There are four components with very clear responsibilities, and each can be scaled out to account for load or geographical considerations.
High-level architecture
Client tools
Client tools are made up of several parts.
- A client library encapsulating common functionality reducing the need to write duplicate code across clients. This also ensures consistent behavior across different client applications, streamlines the development process, and simplifies scalability.
- An independent client application dedicated to offering full functionality around sustainable scheduling. This client will allow system and user configuration in addition to standard scheduling functionality integrated into third party clients like Outlook.
- Plugins for popular applications that are used for the scheduling of meetings – Outlook being a top candidate for integration.
Regional cache and services
The primary role of this component is to enhance performance. Dynamically identifying the best location and time candidates for a meeting involves considering multiple routes for various locations, times, and invitees.
Optimal routes between specific source and destination locations generally remain consistent for a given time unless exceptional circumstances occur, such as an accident or a breakdown of public transport services. Therefore, performing route optimization calculations each time a meeting is scheduled is inefficient. Caching factors such as distance, time, environmental impact, and safety significantly reduces the processing overhead required for searches.
The frequency of refreshing cached metadata used in searches is configurable, allowing for an optimal balance between performance and accuracy. A strategy that combines periodic refreshes with updates triggered by events that may impact routes should keep the metadata sufficiently current to yield accurate scheduling results.
System settings that manage cache refreshing are handled by the Central scheduling services component. This component executes cache updates through API calls to Regional cache and services components, which adjust input parameters based on local user settings.
Central scheduling services
This component is responsible for determining the location and time candidates that best meet the specified criteria. It draws its inputs from the local cache and provides results to the client application. Additionally, it manages system settings to balance the cost of calls to third-party services with the freshness of the cached data.
Process flow
While direct communication takes place between all components of the architecture for reasons including synchronization and currency, the general flow starts with establishing an up to date cache of pertinent routing meta data for each individual including:
- Travel time for time/location pairs
- Preferences for location and time ranges
- Mode of transport availability
- Project membership
- Personal configuration settings
While some of this data is sourced directly from the individual they are associated with, key information derived from routing information is obtained from third party routing services.
Next is execution of the scheduling algorithm in response to either a manual or automated request for a meeting, which utilizes the cached data to produce the results. Then the third step is to visualize these results to the User or Calling process.
Execution flow
Result visualization
Many factors contribute to the optimal satisfaction of criteria for a location and time combination. It's important to present these results in a simple form that allows the scheduler - whether a person or an automated system - to easily identify the strengths of each top location/time candidate. This clarity enables quick determination of the most appropriate candidates for invites or identification of cases where no suitable options exist within the supplied parameters.
Radar graphs, or spider charts, are ideal for displaying multivariate data in a single graphic, providing a comprehensive view of performance across all criteria for each candidate. By using multiple radar graphs, each representing a single location/time candidate, the strengths and weaknesses of each option are clearly visible. This makes it easy to evaluate the trade-offs between different candidates.
Sample visualization of results
A storage framework for caching and calculations
The Apache AGE Extension for PostgreSQL installed on Fujitsu Enterprise Postgres is an ideal solution for the caching and complex scheduling functionalities required in this system, such as identifying the best time slots for meetings among multiple individuals with diverse preferences and constraints.
AGE seamlessly integrates the capabilities of graph databases into the robust and secure Fujitsu Enterprise Postgres environment, providing a hybrid approach that leverages both relational and graph data models. This integration is particularly advantageous for scheduling applications, where relationships and interdependencies between entities - such as people, timeslots, and locations - are inherently graph-oriented.
One of the primary strengths of AGE lies in its support for the Cypher query language, a powerful and intuitive language for graph queries. This feature allows us to efficiently model and query the intricate relationships involved in our scheduling scenarios. For example, determining optimal meeting times involves traversing the graph to find common available time slots that match the preferred locations and time ranges of all participants. The ability to use Cypher within Fujitsu Enterprise Postgres makes it straightforward to express and execute these complex queries, enabling rapid and accurate identification of suitable meeting times.
Furthermore, AGE's seamless integration with Fujitsu Enterprise Postgres means that users can continue to benefit from the advanced features and stability of the latter while adding graph database capabilities. This hybrid app roach allows for efficient data storage, management, and retrieval, combining the strengths of relational and graph databases.
Apache AGE architecture
For our scheduling system, this means that metadata such as user preferences, availability, and location data can be stored in relational tables, while the relationships between these entities are managed using graph structures. This results in a highly performant and scalable solution that can handle large datasets and complex queries with ease.
Approach to utilizing AGE for node and relationship management
Graph structure illustration
Defining the nodes
At a basic level the graph component of our database will contain nodes Nodes represent entities within a domain, and store data as key-value pairs. Each node contains a set of attributes and their corresponding values - for example, a node representing a person might have the attributes id and name, with values that describe those attributes. and relationships Relationships represent a link between a source and a target node, and always have a direction and a type. Like nodes, relationships can have properties (key-value pairs) to provide additional details about them..
The three core node types are:
- Person: Represents each individual
- Location: Represents each location that could be considered for meetings
- Timeslot: Represents time intervals
Person nodes
SELECT * FROM cypher('sustainable_meeting_scheduler',
$$ CREATE (p1:Person{id:'person1',name:'Marcos'}),(p2:Person{id:'person2',name:'Gary''}) $$
) AS(v agtype);
Timeslot nodes
SELECT * FROM cypher('sustainable_meeting_scheduler',
$$ CREATE (t1:TimeSlot{id:'slot1',start:'2024-07-01 09:00',end:'2024-07-01 10:00'}),
(t2:TimeSlot{id:'slot2',start:'2024-07-01 10:00',end:'2024-07-01 11:00'}) $$
) AS(v agtype);
Location nodes
SELECT * FROM cypher('sustainable_meeting_scheduler',
$$ CREATE (l1:Location{id:'loc1',name:'MacquarieOffice'}),
(l2:Location {id:'loc2',name:'Beat Street Cafe'}) $$
) AS(v agtype);
Defining the relationships
The four core relationship types include:
- PREFERS_TIME: Connects a person to their preferred time slots
- PREFERS_LOCATION: Connects a person to their preferred locations
- AVAILABLE_AT: Connects a person to a timeslot they are available at
- LOCATED_AT: Connects a timeslot to a location
Relationships
SELECT * FROM cypher('sustainable_meeting_scheduler',
$$ MATCH (p:Person{id:'person1'}),(t:TimeSlot{id:'slot1'}) CREATE (p)-[:PREFERS_TIME]->(t) $$)AS(vagtype);
SELECT * FROM cypher('sustainable_meeting_scheduler',
$$ MATCH (p:Person{id:'person1'}),(t:TimeSlot{id:'slot1'}) CREATE (p)-[:AVAILABLE_AT]->(t) $$)AS(vagtype);
SELECT * FROM cypher('sustainable_meeting_scheduler',
$$ MATCH (p:Person{id:'person1'}),(l:Location{id:'loc1'}) CREATE (p)-[:PREFERS_LOCATION]->(l)$$)AS(vagtype);
SELECT * FROM cypher('sustainable_meeting_scheduler',
$$ MATCH (t:TimeSlot{id:'slot1'}),(l:Location{id:'loc1'}) CREATE (t)-[:LOCATED_AT]->(l) $$)AS(vagtype);
Defining preferences
We will represent the environmental impact (CO2), safety, and travel time properties associated with the travel from a person's home to a location at a specific timeslot; you can use a dedicated vertex (node) to store these properties. This vertex will act as a hub connecting the person, timeslot, and location nodes.
Vertex in focus in graph structure
Travel time, environmental impact, and safety rating
SELECT * FROM cypher('sustainable_meeting_scheduler',
$$ MATCH (p:Person{id:'person1'}),(t:Timeslot{start:'2024-07-04 09:00'}),(l:Location{id:'loc1'})
CREATE (p)-[:SCHEDULED_AT{travel_time:30,env_impact:'low',safety:'high'}]->(t), (t)-[:LOCATED_AT]->(l) $$
) AS (v agtype);
SELECT * FROM cypher('sustainable_meeting_scheduler',
$$ MATCH (p:Person{is:'person2'}),(t:Timeslot{start:'2024-07-04 10:00'}),(l:Location{id:'loc2'})
CREATE (p)-[:SCHEDULED_AT{travel_time:45,env_impact:'medium',:'medium'}]->(t),(t)-[:LOCATED_AT]->(l) $$
) AS (v agtype);
During development of a prototype the source location could be stored as a property of the person node, and then extended to supporting different source locations at specific timeslots and multiple transport modes via additional relationships between the existing nodes. The source location is not shown in the diagrams to reduce complexity and is not strictly required in this part of the architecture (only required as a parameter to the route optimization services to calculate the cache data).
Querying the graph database structure
The main query aims to find the best timeslot and location pairs for multiple persons by considering their preferences and availability, along with the optimal travel details. We can construct a query that does the following:
- Match persons and their preferences
Match Person nodes with their preferred locations and available timeslots.
- Match travel details
Match the SCHEDULED_AT vertex to find the travel times, environmental impacts, and safety ratings associated with the tri-node relationship of person, timeslot, and location.
- Filter and aggregate
Filter the results based on the desired criteria (e.g., lowest travel time, best safety rating) and aggregate the data to find the optimal timeslot and location pairs.
Example Cypher query
SELECT * FROM cypher('sustainable_meeting_scheduler',
$$ MATCH (p:Person)-[:PREFERS_LOCATION]->(l:Location),
(p)-[:AVAILABLE_AT]->(t:Timeslot),
(p)-[:HAS_TRAVELDETAIL]->(sa:SCHEDULED_AT)-[:AT_TIME]->(t),
(td)-[:TO_LOCATION]->(l)
WITH p, l, t, sa
ORDER BY sa.travel_time ASC, sa.env_impact ASC, sa.safety DESC
RETURN p.name AS person, l.name AS location, t.start AS start_time,
t.end AS end_time, sa.travel_time, sa.env_impact, sa.safety $$
) AS (person_name agtype,
location_name agtype,
start_time agtype,
end_time agtype,
travel_time agtype,
env_impact agtype,
safety agtype);
Graph structure efficiency
The main query leverages the graph's pre-established relationships, allowing for efficient traversal and filtering. Instead of performing multiple joins and searches typical of relational databases, the graph query directly accesses and orders the interconnected nodes and properties, resulting in faster execution and real-time decision-making.
This method is particularly efficient because it reduces the computational complexity by taking advantage of the graph structure where relationships are explicitly defined and indexed, minimizing the need for extensive searches and joins.
This approach is ideal for scenarios such as this which involve complex, interconnected data where optimal paths and properties need to be evaluated quickly.
Conclusion
The rapid shift towards remote and hybrid working models has fundamentally transformed organizational operations, creating both opportunities and challenges. The logistical complexity of coordinating face-to-face meetings and on-site collaborative activities across various locations and time zones necessitates sophisticated solutions. My proposed system addresses these challenges by leveraging advanced route and scheduling optimization techniques, enhanced through Graph Database technology. By integrating diverse data sources and services, the system intelligently evaluates and optimizes travel and meeting logistics, ensuring efficient and effective collaboration.
A cornerstone of this optimization framework is the use of graph databases, which excel in modelling and analyzing complex relationships and networks. Graph databases not only facilitate the calculation of the shortest paths but also evaluate various relational aspects such as attendee availability, cost constraints, and transportation modes. This allows for nuanced recommendations that align with organizational goals and constraints. The inherent efficiency of graph databases stems from their ability to directly access and traverse pre-established relationships, avoiding the computational overhead associated with relational database joins and searches.
Through the comprehensive exploration of remote and hybrid working challenges, the presentation of a robust route optimization system, and the pivotal role of graph databases, this paper underscores the potential for enhancing the efficiency and effectiveness of logistical planning. The integration of these advanced technologies promises a cohesive solution that supports sustainable and user-friendly scheduling practices, ultimately fostering a more dynamic and adaptable collaboration ecosystem.