Fujitsu is at the forefront of addressing these challenges, ensuring that the organization’s data remains protected through advanced security technologies.
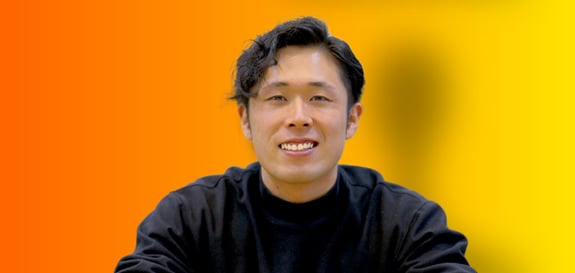
Expansion of in-house data utilization for business specific generative AI
As organizations increasingly leverage their in-house data for generative AI, the associated security risks also escalate. Traditional generative AI models primarily utilized publicly available data. However, the latest trend involves incorporating proprietary data, which necessitates robust security measures at the database layer to prevent data breach¬es.
Tailored advisory services for IT planning in the banking sector, specialized clinical assistance with deep clinical knowledge, and corporate analysis for individual investors using reliable data from reviews are some of the use cases of today’s generative AI. These examples highlight the shift from using publicly available data to integrating specific in-house data.
Growing security risks with data utilization
Traditional generative AI models, which learn from publicly available data, struggle to provide accurate responses to industry-specific queries. RAG (Retrieval-Augmented Generation) enhances these models by integrating related information from a vector database, significantly improving the accuracy of responses to specialized questions.
While RAG improves response accuracy, it also introduces new security risks, particularly in managing the vector database that stores proprietary data. Ensuring the security of this database is crucial to prevent unauthorized access and data breaches.
Key database security risks for RAG
- Misconfigured access permissions - Errors in access rights can lead to data leaks.
- Prompt injections - Unauthorized data exposure through malicious queries.
- Data tampering - Altered data leading to incorrect AI-generated responses.
Misconfigured access permissions
Adjusting access rights is essential when using in-house data. Properly configuring these rights can be com¬plex and error-prone, potentially leading to unauthorized data access. Ensuring meticulous design and implementation of access controls is critical.
Prompt injections
AI systems can be manipulated through malicious queries that bypass standard security protocols. This is known as prompt injection attacks. Implementing robust input validation mechanisms to detect and block such queries is essential to protect sensitive information.
Data tampering
Unauthorized modifications to data within the vector database can result in incorrect responses from the AI system. Implementing measures to detect and prevent data tampering is crucial to maintain the integrity of the AI-generated outputs.
Comprehensive database security measures for utilizing in-house data
Although a layer of security measures can be taken at the AI tooling and application level, database layer security is critical to protect your organization’s data assets. The complexity of managing access rights and the high variability of natural language inputs pose significant challenges, demanding a robust database system built on a foundation of security.
Access permissions management
Fujitsu Enterprise Postgres simplifies access control with the Confidentiality management feature by managing permissions directly within the database, reducing the risk of configuration errors and ensuring that only authorized users can access sensitive data.
Data anonymization
Implementing data anonymization techniques helps protect sensitive information from exposure. By hiding or anonymizing data before it is handed over to the LLM process, the risk of data leaks is minimized. Fujitsu’s data masking feature allows flexibility in the masking policies; for example, the columns that are not relevant to the accuracy of LLM processing can be masked completely while others are left anonymized.
Tampering detection and audit logs
Fujitsu Enterprise Postgres includes mechanisms for detecting data tampering and maintaining comprehensive audit logs. These features enable organizations to monitor access and modifications to their data, ensuring any unauthorized changes are promptly identified and addressed.
By working with ScalarDL, non-tamperability is guaranteed, and tampered data will be detected when fetching data. This means compromised data will not flow to the AI system, completely preventing incorrect responses. In addition, the dedicated audit logs include information to help identify exactly when and what manipulation was conducted, in case of unauthorized access.
Strong password policies
Enforcing strong password policies further enhances security by preventing unauthorized access to the database. Fujitsu’s policy-based password management restricts access to accounts at the database layer instead of application layer by setting password restrictions and establishing rules for password complexity and preventing unauthorized logins.
Fujitsu Enterprise Postgres comes with enhanced security measures described above, with no extra cost to future proof your data with security and reliability.
Conclusion
Fujitsu’s advanced security technologies provide robust protection for confidential data amid the increasing use of generative AI. By integrating comprehensive security measures within the database and utilizing innovative techniques like RAG, Fujitsu ensures that organizations can leverage their in-house data for AI applications without compromising data security.